Predicting churn is vital for subscription-based businesses. Here's a simple guide to figure it out.
Customer churn is a part of business. It’s never easy, but it teaches us about trust, loyalty, breaking points, and out-of-our control circumstances that lead to someone leaving.
But for subscription based businesses, customer retention is a primary KPI, and the market is ripe with competitors. Subscription-based businesses are always one bad experience away from losing even the most loyal customers.
But what if you can predict a customer leaving and figure out what you can do to stop them from leaving?
Customer Churn Definition
Real quick, let’s define what customer churn is:
Customer Churn: When a customer ends their relationship with a company, product or service. This could be in many different capacities, such as website churn, subscription churn, newsletter churn, content churn, etc.
Churn Rate: The percentage or ratio of users who churn within a time period. This is important to measure because you can use this data to identify trends and relationships between variables. Learning about and dissecting your churn rate is the key to developing customer retention and which customers to put your time and resources into. The churn rate impacts the bottom line and can make it or break it for businesses.
Typical causes for customer churn can be:
- Poor customer service
- Customer found an alternative solution
- Not enough value
- Failure to meet market quality
Churn negatively impacts every area of a business. When your customers churn, you’re not only losing money, you’re losing market share to your competitors.
Why Predicting Churn is So Important for Subscription-Based Businesses
Predicting customer churn is a challenging, and incredibly important, business problem, particularly for subscription-based businesses. Most SaaS and telecom products are subscription based, meaning the company's revenue depends on how many users are paying monthly, weekly, or yearly.
In these kinds of industries, the cost of customer acquisition is high. Having the ability to predict when a particular customer is at risk of churning (while you can do something about it) can help keep that cost down.
Using your data, you can detect relationships between a user churning and your product attributes. This is an amazing ability for product managers, sales teams, and designers, but especially useful for customer success managers: this team can proactively identify and engage with a customer who is at risk of churning.
Related reading: How to Market to Your Users During a Quarantine
So, if you know what to look for, and what numbers to crunch, you can prevent customers from leaving. And that small act adds up. According to research conducted by Bain and Company, a mere 5% increase in retained customers and avoiding churn can increase your profits by 25%-95%.
The problem is: not everyone has access to this kind of data. And if they do, they don’t always have the technical expertise to apply predictive analytics.
No-code Machine Learning and Churn
This is where no-code machine learning tools are changing the game.
All you need is a tabular dataset with quantitative information (CSV file, or data source such as Salesforce, Hubspot, etc.) about your customers and in just a few clicks, you can create a model that will predict their likelihood of churning.
With this kind of power, you can predict what your churn rate will be, but also identify who might leave your company.
Traditionally, data science teams would conduct all these steps manually. It’s a time consuming process that requires highly technical skills. The kind of insights you need to quickly see and understand who might churn would get buried in a bottleneck.
But no-code machine learning removes all that bottleneck by putting the power of predictive analytics into the hands of everyone. This means that a customer success manager could quickly generate a model to understand how at risk a customer is of leaving, in just minutes.
With that kind of knowledge, they can engage with customers at pivotal moments to increase retention and prevent loss of revenue.
Related reading: Reasons to Master No-code Machine Learning
What Kind of Data Do You Need to Predict Customer Churn?
As mentioned, you want to measure churn rate, but also variables you can easily track that might attribute to churn.
In one of our first posts on an introduction to creative data predictions, we outline the data prediction process. The first step is defining a business problem.
In this case, the business problem can be:
How do we identify which high-value customers will churn and what attributes affect them churning?
Beneficial Data Attributes to Collect for Churn
Let’s list out valuable data points.
We can largely classify a dataset into five different sections:
- Identifying Data: This can be a unique customer ID, name, or code.
- Demographical/Basic Customer Data: Basic info about the customer such as age, income, education, location, etc.
- Product Data: The customer's usage of the service such as type of plan, tenure, number of products used, etc.
- Support Data: Info on how the customer interacts with customer support, number of interaction, topics asked, and satisfaction ratings.
- Payment Data: Quantitive data on how much the user is spending on their plans, their monthly spend, weekly spend, micro-transaction data, etc.
Other contextual attributes that would be interesting to discover relationships between are:
- Overdue balances
- Credit score
- User income
- Company title
- Device type
- Time they use the product
Exploring Why Customers Churn With Sample Data
Sometimes the conversations you have about your data is hard and can uncover some ugly truths.
In the technical machine learning realm, the process would be to prepare and clean data, build a Logistic Regression model, train it and test it, and then start making predictions.
However, we’re going to take a simpler approach where you can click a few buttons.
Going back to the problem, Input this question: "Which customers will churn and why?" Let's explore the results of a codeless data prediction as it relates to churn.
When you login to Obviously AI, you can do some amazing things in under a minute.
- You can use sample data inside our data store to model your dataset after.
- You can predict churn with sample AT&T telecom data to get an idea of the type of attributes related to churn.
- You can use your own data to make predictions related to churn.
We'll use sample data to use as an example.

Simply choose the column "churn" and hit Go.
You can customize your prediction and filter out columns you don't want to use. For this prediction, the identifier column is the unique user ID because you want to identify which users are most and least likely to churn. The prediction column is, obviously, Churn.
If you wish, read more on how our no-code algorithms work after you start predicting. Each algorithm is trained, tested, and customized in under a minute.
Let's start predicting.
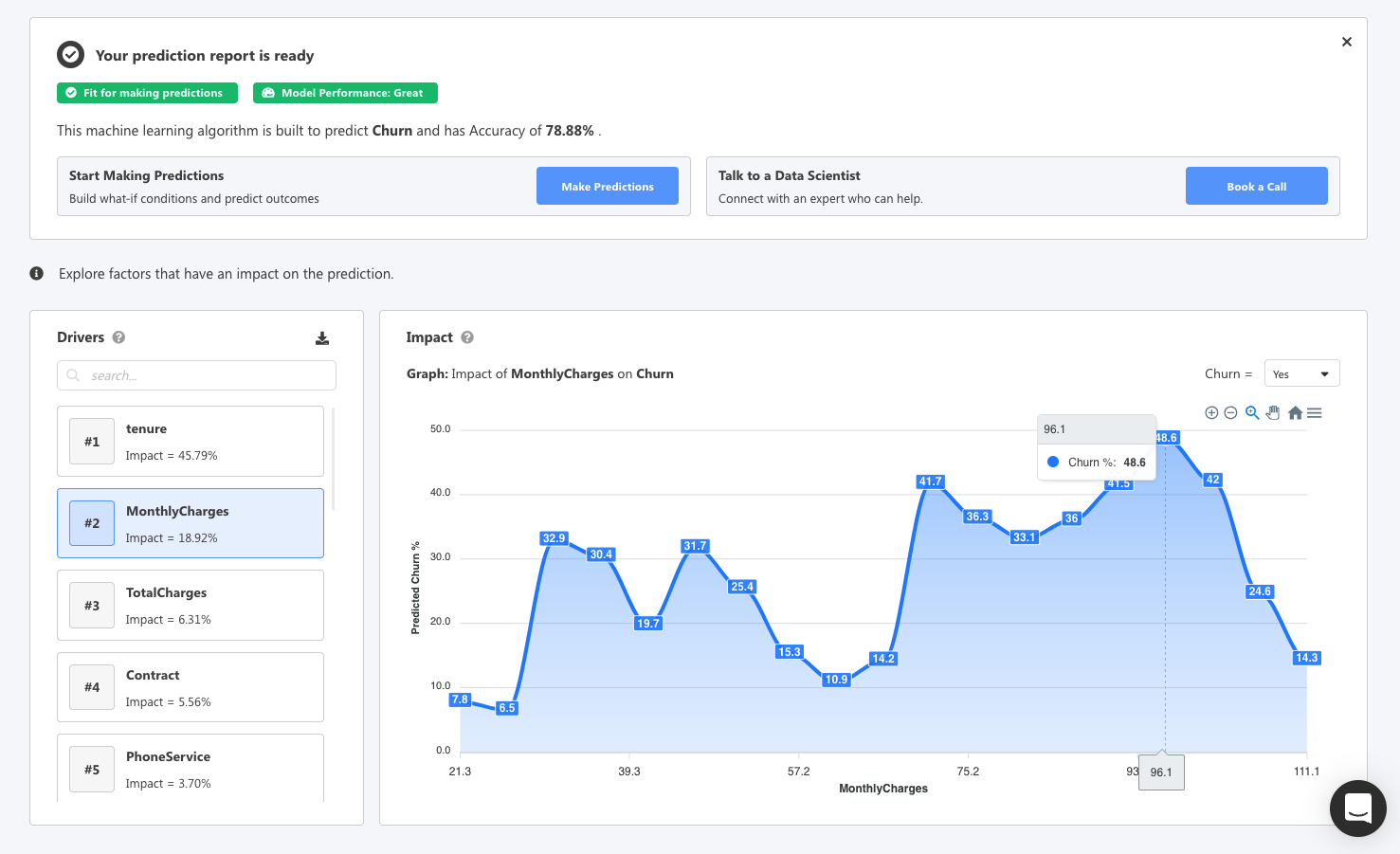
Instantly you can see the top drivers related to churn such as tenure, monthly charges, total charges, type of phone service, and more.
Using the interactive graph you can see the predicted churn related to the attribute. For the image above, customers with a monthly spend of $96.10 have a 48.6% chance of churning.

You can start to explore the top drivers to improve the customer experience. Here you can see customers are more likely to churn if they’re on a month-to-month contract.
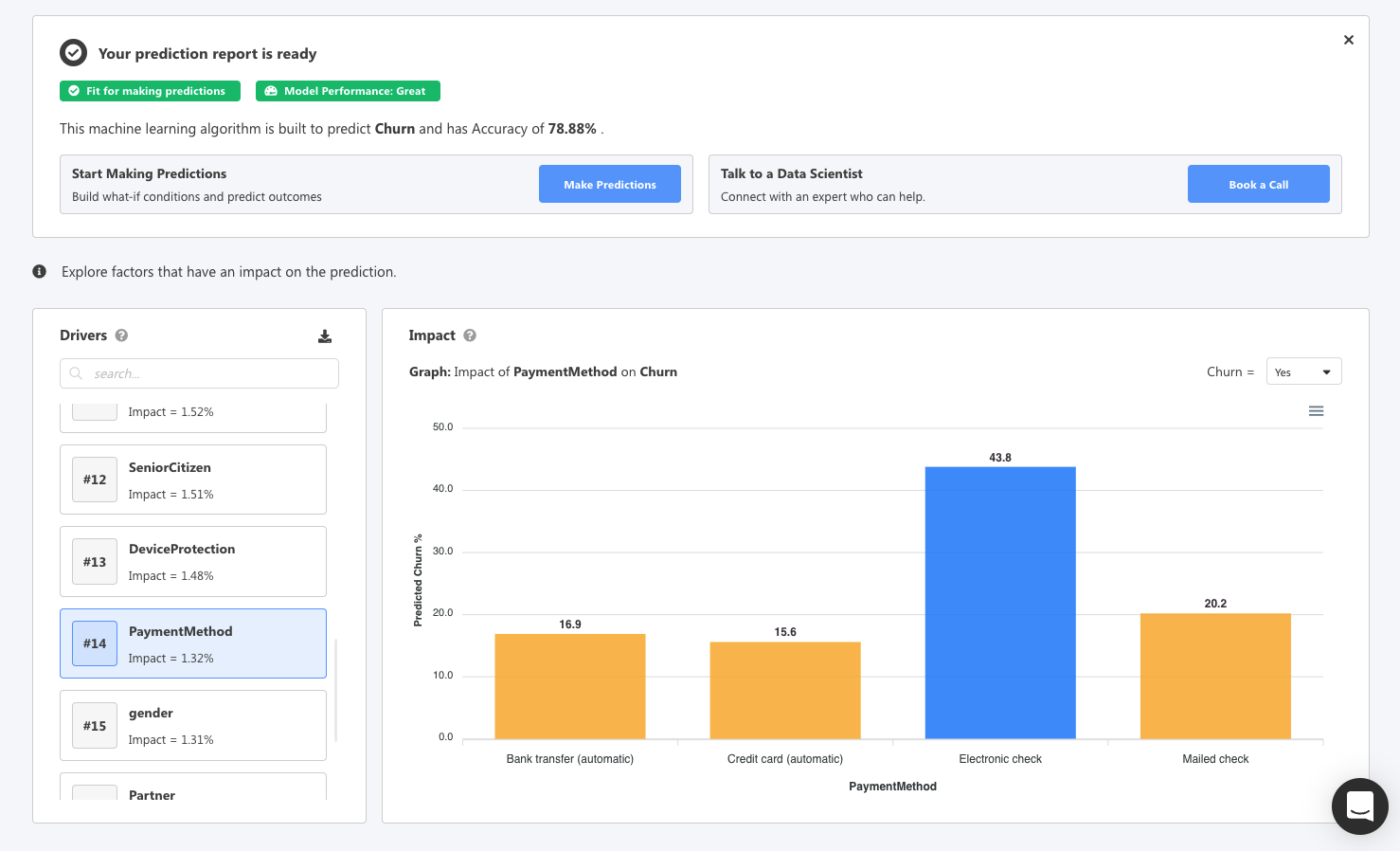
Diving into the deeper details, you can see how payment methods compare to each other when it comes to churn. You can quickly see those who are paying by electronic check are more likely to churn. This can mean the payment process for electronic check is difficult and your product can be geared towards the user using automatic payments instead to increase retention.
Having this kind of knowledge can be a complete game changer. With insights into demographics, time, product engagement, etc., you can really dial into preemptive retention strategy efforts. The best part? These predictions can be fed directly into your CRM systems in real time!
Customer Retention Remains One of the Biggest Problems in Business
We’ve only just scratched the surface—there’s so much you can do when you have the power of predictive analytics on your side.
While churn is a hard egg to crack when improving a product, you can use historical data to predict the best ways to improve retention and allocate resources. You can even see how many users will churn down to a specific date, like by the end of the month or quarter—read our blog about time series to see this in action.
Machine learning predictions gives you answers instead of moving blindly into product optimization. And codeless predictions empower non-technical teams to quickly get insight and take actions from their predictions.
Want more? Take a look at our churn prediction case study.