Employee attrition used to be a mystery. Here's how you can unlock insights with AI.
The Human Resources (HR) department is in charge of taking care of employee’s well being and ensuring they are happy with their position. With machine learning, you can actually predict employee attrition to see what causes a valuable employee to leave or stay with a company. This is perfect for HR managers planning their hiring and auditing employee experience.
What is Employee Attrition?
According to Jobzology, “Staff attrition refers to the loss of employees through a natural process, such as retirement, resignation, elimination of a position, personal health, or other similar reasons. With attrition, an employer will not fill the vacancy left by the former employee.”
Attrition is different from turnover. Employee turnover refers to an employee being terminated or resigning with the purpose of being replaced. Attrition occurs when the role is left vacant or eliminated.
A major challenge that businesses face today is the problem of understanding and predicting which employees are going to leave. If you don't have a good understanding of your staff or you aren't aware of some helpful tech-based tools that are at your disposal, predicting attrition is tough.
Reasons to Predict Employee Attrition
Employees are not always going to participate in the offboarding process, or they might not be forthcoming in their HR exit interview. And by the time the exit interview comes around, it’s too late to address issues that caused that employee to leave in the first place.
In fact, according to the Work Institute’s 2020 Retention Report, 78% of the reasons employees quit could have been prevented by the employer.
Attrition is a major cost for any organization. According to the Center of American Progress, predicting turnover would help save money in the long run.
“For positions that earn between $30,000 and $50,000 per year, the cost of replacement was found to be 20% of annual salary. For executives earning high salaries, the cost of replacement was found to be 213% of annual salary. An executive who earns $100,000 would cost $213,000 to replace.”
With less skilled workers available to do the work, it becomes harder to get the results needed to keep profits up and morale high. As well, what’s lost when a productive employee quits isn’t just the person or the cost of their replacement. It’s the new product ideas, great project management, customer relations, and more.
Predicting turnover helps you understand which employees are at risk of leaving and get a hint of what interventions could be implemented to reduce the change of attrition.
With machine learning, HR managers are able to see not just to see what happened, but understand why it happened, what will happen next, and how to adapt their workforce strategy to align with company objectives. This helps HR to foresee vacant positions, team budget needs, what employee benefits they can improve to keep employees happy, what departments are the most and least likely to stay at a position for a length of time, and more.
As a business, you can predict questions like:
- What causes employee churn?
- Why do we lose valuable employees?
- When will an employee most likely leave the company?
Most organizations have a gold mine of company data. And when this data is leveraged with no-code machine learning tools to gain valuable insight about staff, you can be proactive and manage potential issues before they arise.
Let’s explore how to predict employee attrition with machine learning.
About the Dataset
We’re using a sample dataset from our Obviously AI Data Store. It’s also available to download from Kaggle.
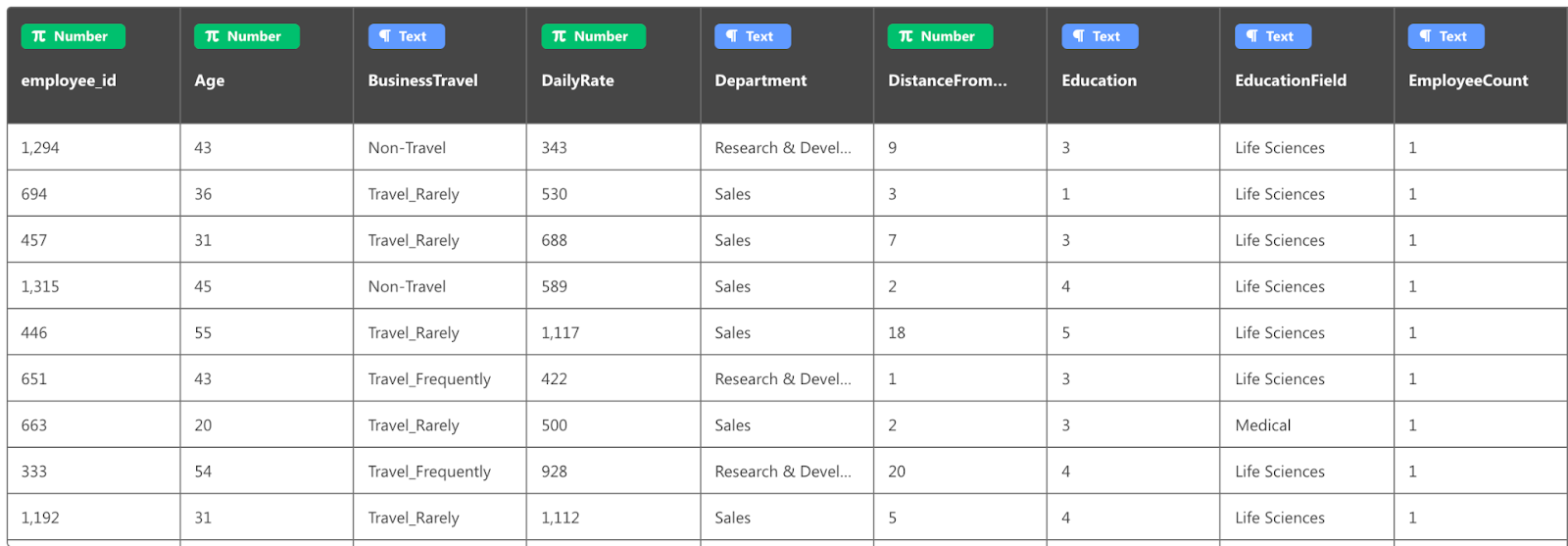
In the dataset we’re using, every single row is an employee, and every column tells us something about that employee, such as age, education, and their tenure at the company.
The column “Attrition” which tells us if the employee in that row is still with the company.
Using this historical dataset, we can begin creating our model to help us predict whether a current employee will leave the company or not.
Exploring the Results of the Data Predictions
If you’ve been following us for a while, you know we’ve simplified the machine learning process down to 3 easy steps. You can compare it to a more complex process and see the benefits of no-code machine learning compared to traditional.
When you work in Obviously AI, all you have to do is bring your historical data. And in just a few clicks, you can start predicting - Obviously AI will automatically begin building a machine learning model to make predictions—all in a matter of seconds.
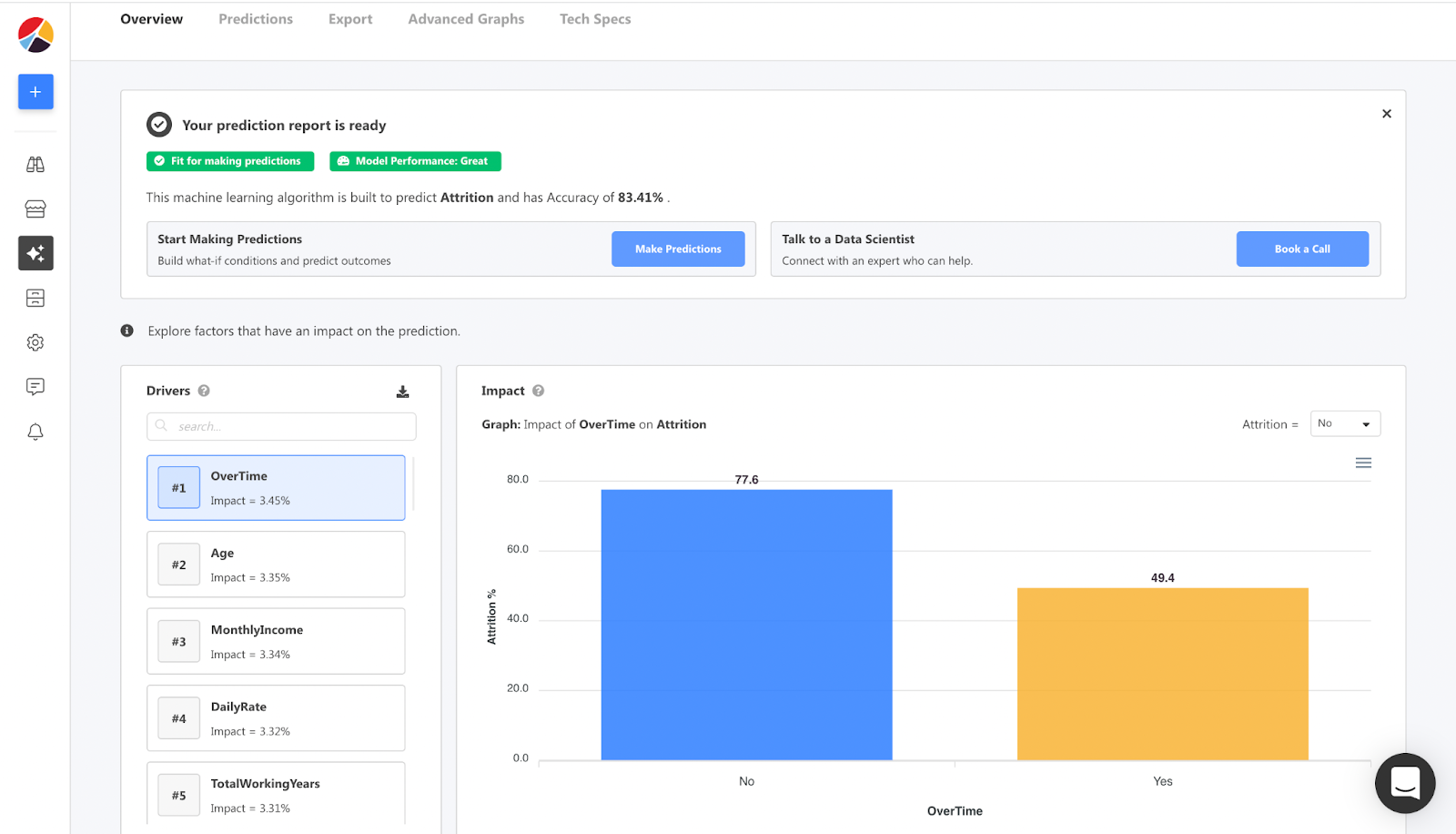
Related Reading: Reasons to Master No-code Machine Learning
In our Prediction Report, we can see the top drivers related to employee attrition:
- Overtime
- Age
- Monthly Income
- Daily Rate
- Total Working Years
Our top driver is overtime. And if we dig a little deeper, it looks like when an employee consistently works overtime or is given the overtime option, they have a 50.6% chance of employee attrition, compared to 22.4% chance of not leaving.
Let's take a look at another driver—Age.
According to ZipRecruiter, early stage employees should aim for a promotion around every year. If we click on the ‘predictions’ tab, we can toggle all sorts of filters for ‘what if conditions’—this is a way to understand what kind of business impacts can arise from changing one or more variables. We can play around with different value combinations to ask different questions about our employee attrition data.
Each time we make a selection, the combination is assessed and a prediction is generated—instantly.
This graph looks right on the money.
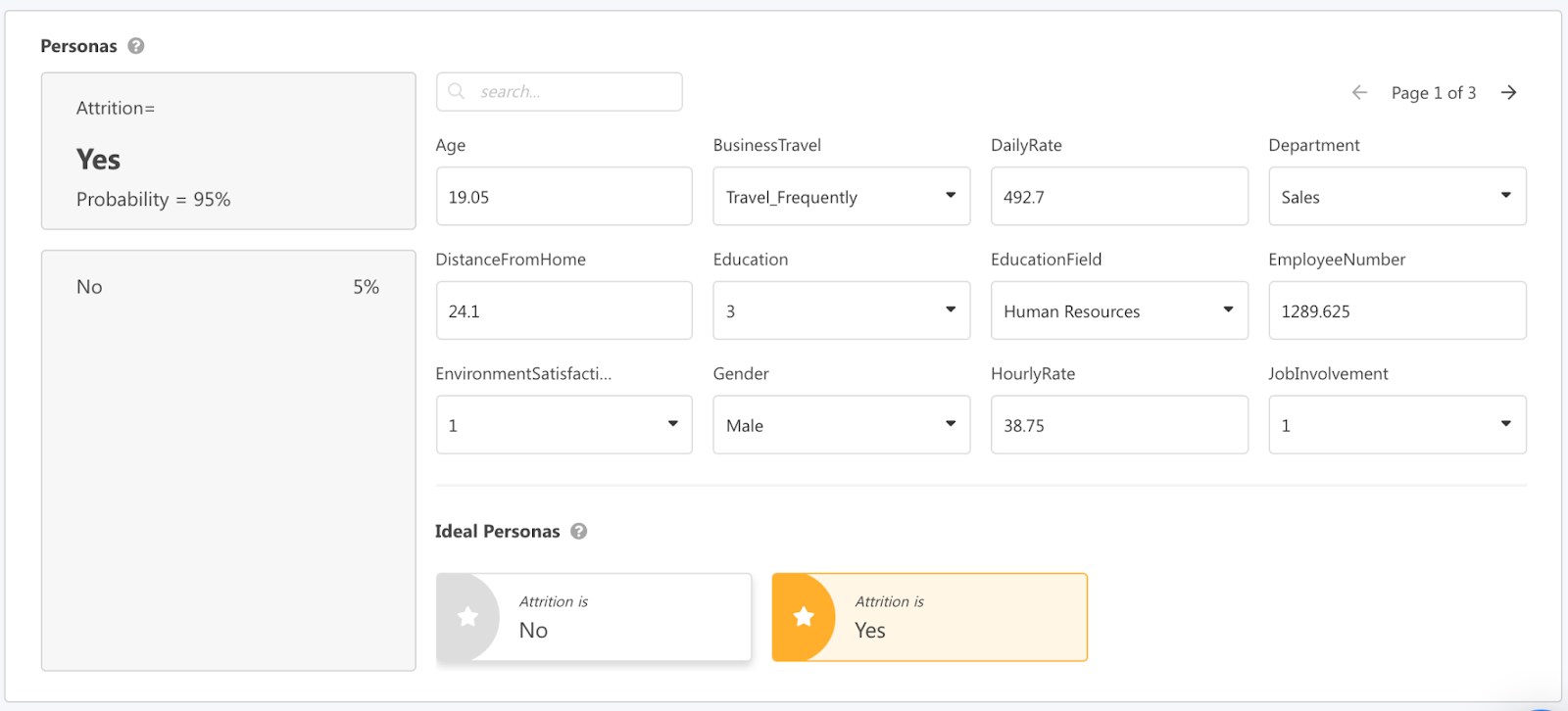
If we want to understand the likelihood of a young professional, who is at least 19 years of age, has worked at this fictional company for four years, been in the same position for 3 years, and had no promotion, we can see that they are 95% likely to leave the company.
We can also explore drivers we're interested in learning more about. For instance, let's say we want to know which department is the most unsatisfied.
If we toggle that driver, it looks like the Sales department at this fictional company has a higher chance (37%) of employee attrition, compared to R&D and HR.
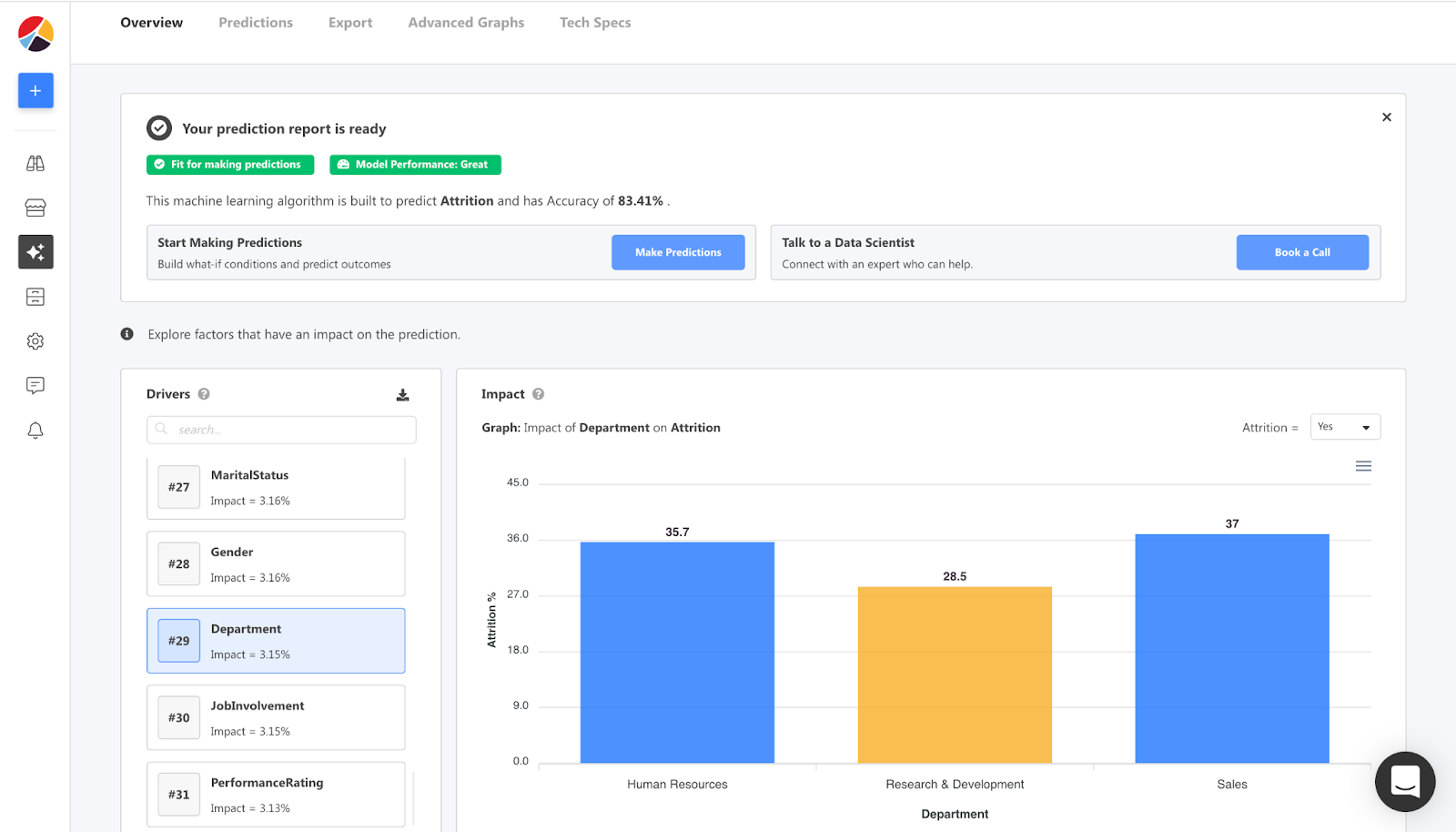
How Predicting Employee Turnover Helps
We’ve only scratched the surface. The benefits of applying an employee turnover prediction model like this extend beyond its pure prediction capabilities. It gives us insights that can modify operations of an organization as a whole.
For instance, we now know that:
- Sales has a 37% of employee attrition, comparatively higher than other department. This tells us our retention efforts should prioritize Sales.
- Age has a huge impact on whether an employee remains at a company or not. When we compare this data to years since last promotion, we get a fuller picture of why.
- Monthly income also plays a major role in whether an employee leaves the company or not.
In terms of cost savings, HR teams can use the model’s explanations to develop retention policies for those departments they’ve deemed at risk. They can also target high risk employees with retention initiatives.
Now, more than ever, business leaders need strategic insight and the ability to model how turnover trends impact revenue and profits—quickly and accurately. Platforms that empower teams to do this in minutes not only have their finger on the pulse of their company, they can proactively take action to reduce employee attrition.
Deliver the Right Retention Results for Your Business
Doing the kind of analytics needed to effectively reduce employee turnover can’t easily be accomplished on multiple spreadsheets. An investment in the right no-code machine learning solution is the key to achieving this.
With the right insights, you can better understand and take evidence-based corrective actions. A no-code machine learning solution like Obviously AI can help you reduce unwanted turnover, focus on top talent, critical positions, successors, and other “must keep” groups.
To learn more about how to leverage no-code machine learning to reduce turnover, be sure to check out our employee attrition case study.
And be sure to read our Ultimate Guide to Machine Learning to get a comprehensive deep-dive on the subject.