Understand your customers better than ever with no-code AI.
In 2020, things are unpredictable. Our time in quarantine—unpredictable. Our yearly travel plans—unpredictable. HBO's new series, Lovecraft Country—unpredictable. Your customers—very predictable.
Where going to do a pop quiz with one question:
Who's the ideal user and how do you know what they will do?
If you're an avid reader of these posts, you know the answer is always the same to these questions.
Obviously, the answer is data. You can use historical data to identify your users with high lifetime value (LTV) and actually predict what they're going to do with our "Personas" feature.

Here’s a guide to create user personas to find users that will have high lifetime value.
Identify What Customers You Want to Find
Generally, businesses want to find and retain the customers that grow their business.
For example, the chat company, Slack, might want to use their data to predict the customers most likely to add teammates to their workspace because when teammates are added, their audience and customer base grows.
Other SaaS products might want to predict which customers will make an in-app purchase and market to them accordingly. Healthcare companies might want to build personas on who is the most likely to show up for appointments.
The measure of success and what attributes to recurring revenue is different for each company.
So, How Do You Identify These Customers From Your Dataset?
There are many creative ways to identify these customers. The easiest way to identify high-value users is to predict the three metrics: LTV, Churn, In-App Purchases. Then, build personas around customers who have the lowest and the highest probability to perform an action.
In our platform, we have preloaded sample data along with our new Data Store, where you can use datasets applicable to your industry for free.
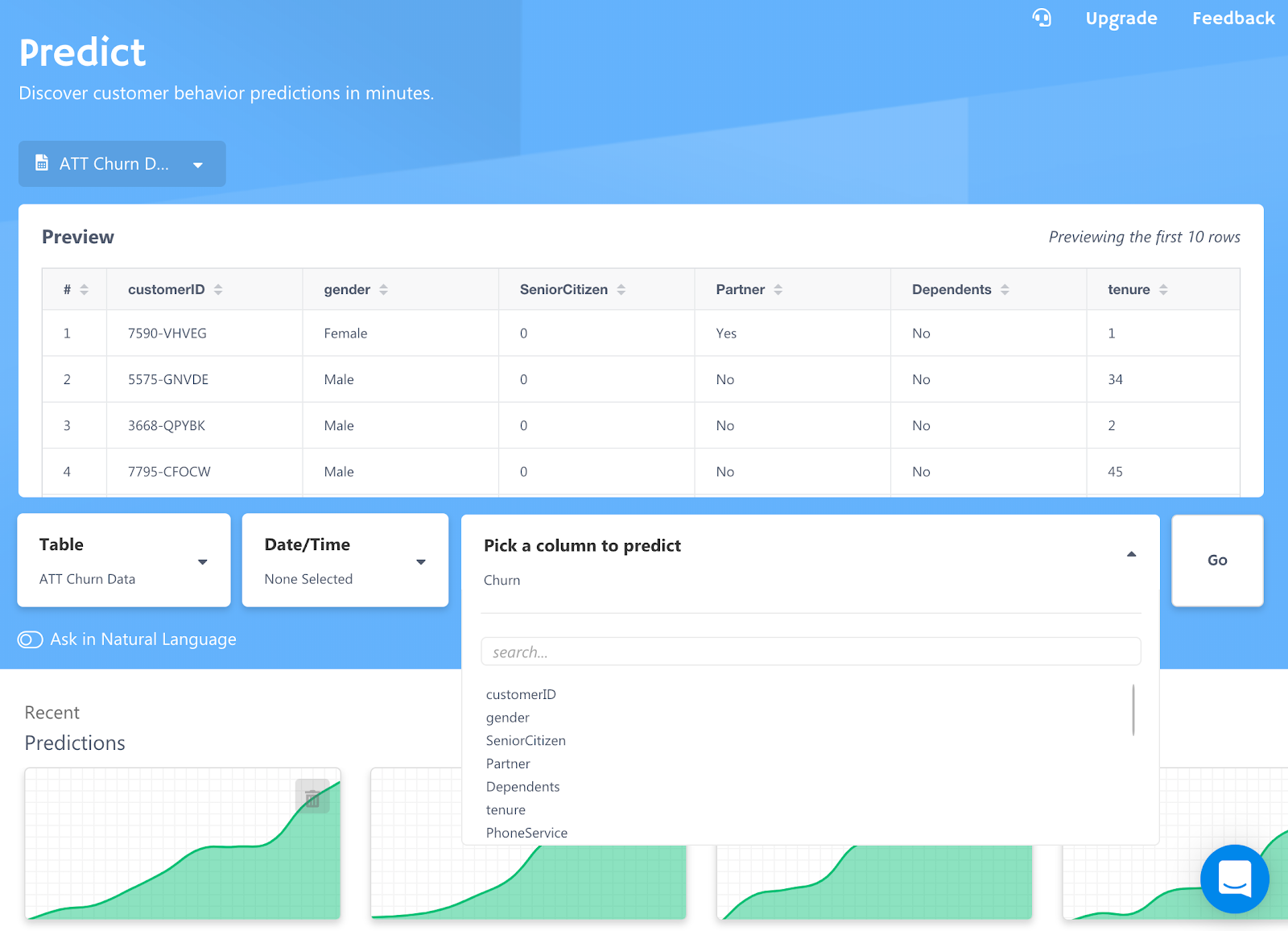
For example, let’s predict customer churn. We touch upon churn a lot in our blog. If you’re interested in learning more about churn, here’s a great post we wrote.
Once you hit “Go,” you will be given the top drivers by impact. Below the prediction report name, click the “Personas” button.
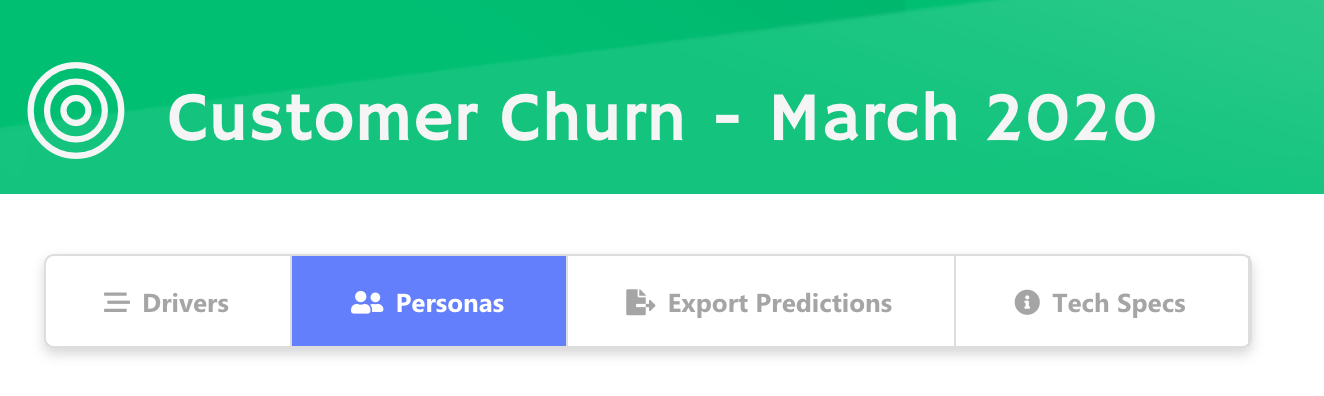
Okay, now you’re on the Personas page.
From here you can build out a persona and see the probability of them churning based on their attributes.
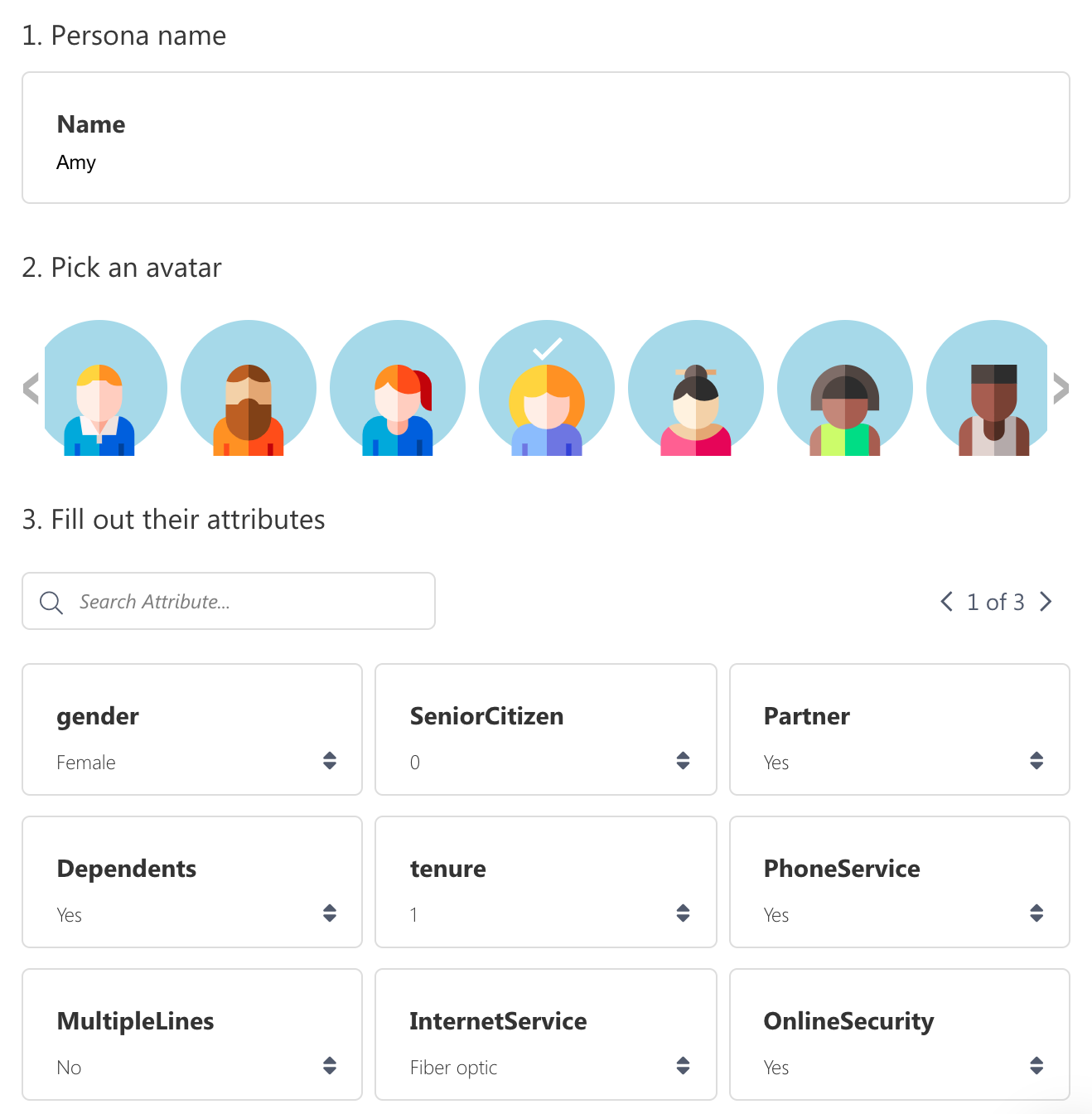
As an example, I built a persona of a female named Amy, based off of Florence Pugh’s character in Greta Gerwig’s Little Women.
Once you have selected the attributes, you’ll be able to see the likelihood of them churning.
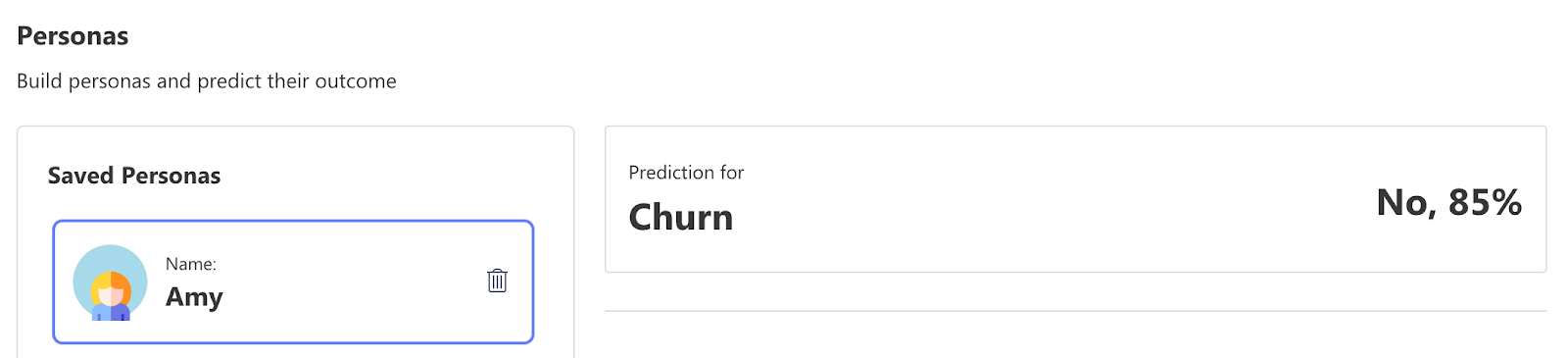
With these attributes and the assigned algorithm, we can see Amy has an 85% chance of not churning.
We can do the same thing with add-ons—or for SaaS—something like in-app purchases, for example. You just follow the same process as you did with Amy. This time, we will measure the likelihood of Timothée Chalamet’s character, Laurie to add multiple lines to his plan.

With the attributes, we can save this persona as someone who definitely wouldn’t add multiple lines to his plan. Marketers and sales teams can use this to better target personas who are more likely to add multiple lines to their plans.
Some other ways you can explore how your users spend is predicting something like Total Charges.
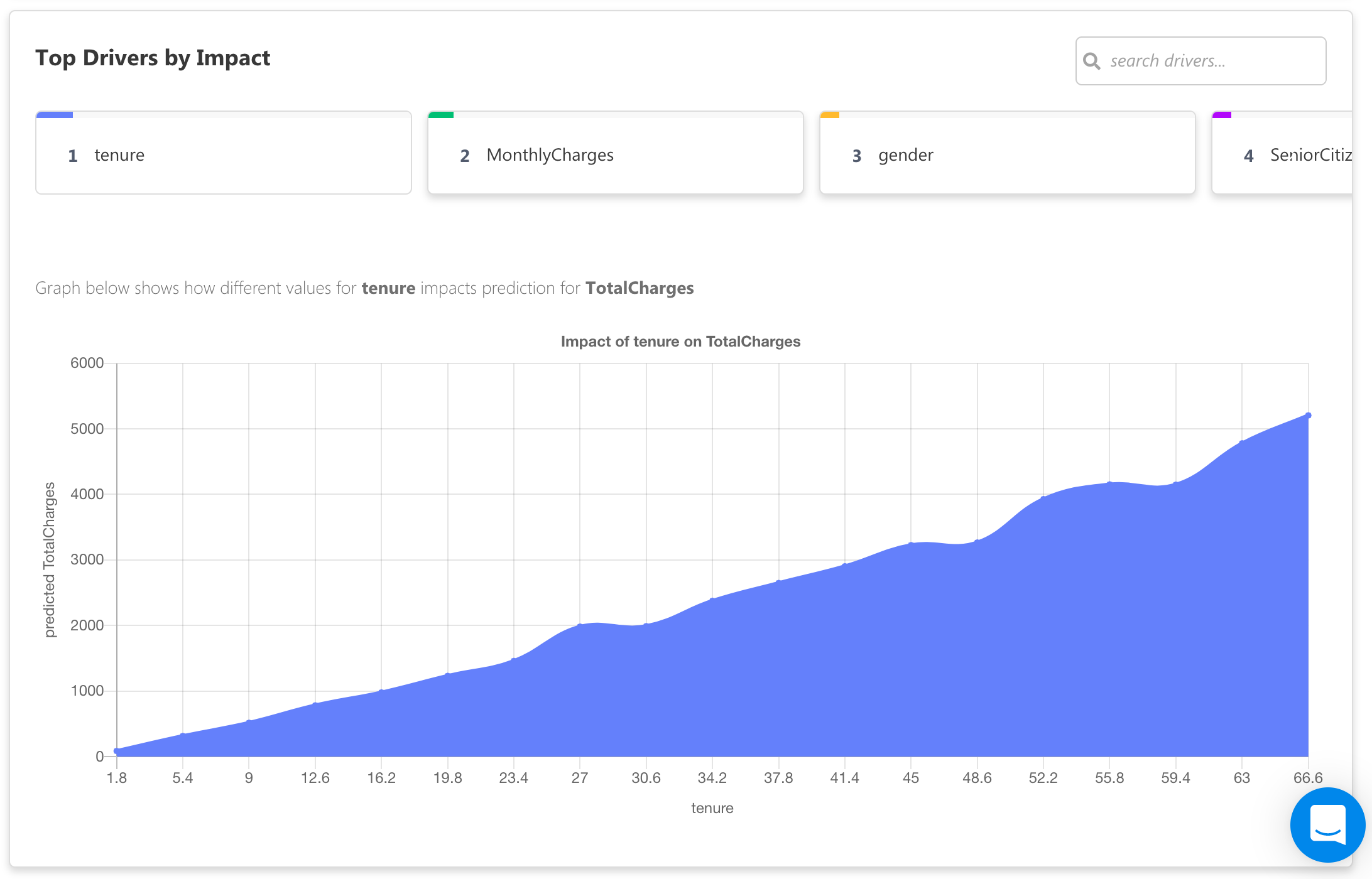
Here you can see the predicted charges in relation to tenure and other attributes and export them to a spreadsheet to share with your team. This way you can see each prediction on an individual level for each unique customer ID.

You can find the Export Predictions button at the top of your prediction report. Don't worry, an export at weblink feature is coming soon so you can share your prediction report via desktop or mobile!
Some More Tips on Building Personas With Machine Learning
Give the persona a name
This makes them easily recognizable and organized. You also want to make the persona feel like a real customer. You want to be able to see your product from their perspective.
Make some attributes the same
When comparing personas, you want to make some attributes the same across the board with one or two different attributes so you can accurately compare them. If two personas have completely different personas, it’s hard to get insights on the differentiating factors between them.
Creating Personas Will Make You a Better Product Builder
With personas, you can hyper-target users and create personalized messaging for them and improve their overall customer experience.
Creating personas has a lot of applications, but starts with data collection. We’ve seen our users use machine learning to measure UX effectiveness, product design, marketing campaigns, and sales campaigns.
Begin using personas with Obviously AI.